How generative information retrieval is reshaping search
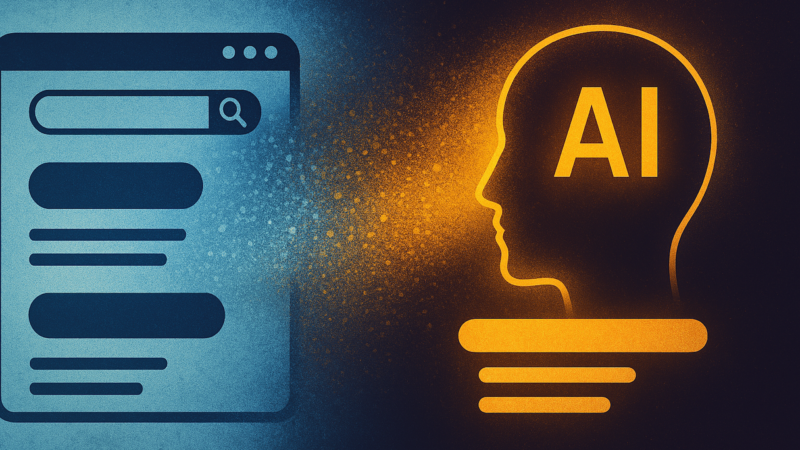
Search is dead, long live search!
Search isn’t what it used to be.
Search engines no longer simply match keywords or phrases in user queries with webpages. We are moving well beyond the world of lexical search, which is simply text-based with no understanding of the semantic connections between not only things but multimedia representations of things/concepts.
Today, AI can understand, contextualize, and generate information in response to user intent largely utilizing probabilistic prediction and pattern matching.
This transformation is being driven by generative information retrieval.
Generative information retrieval is a fundamental shift in how systems surface and present information.
Marc Najork, a distinguished scientist at Google DeepMind, laid out how large language models (LLMs) are changing search and information retrieval during a keynote at SIGIR 2023 that’s worth revisiting. His presentation also explored how we have reached this position via iterative change from lexical to semantic, hybrid, and generative approaches over time.
From retrieval to generation
For decades, search engines have responded to user queries by pointing to documents that might contain the answer.

But that model is evolving. We’re now in the early days of generative information retrieval.
The system doesn’t just find content; it generates answers based on what it retrieves in an increasingly multimodal manner, pulling together everything that an under-specified query might possibly represent, synthesizing in one view.
Najork described this shift as moving from traditional retrieval-based systems, which return a ranked list of documents, to retrieval-augmented generation (RAG) systems.
In a RAG setup, a model retrieves relevant documents from a corpus and then uses them as grounding knowledge and context to generate a direct, natural-language response.

Put simply, searchers aren’t presented with a list of links to webpages. They’re getting synthesized, direct answers, often in the tone and style of a helpful assistant.
This new approach is powered by LLMs trained on vast amounts of data and can reason across retrieved content.
These systems are imperfect. We know they hallucinate and get facts wrong.
We can see for ourselves the many ways in which search engines and other technology companies utilizing AI and large language models, for example, to summarize news headlines and summaries, are struggling to control the hallucinatory nature of LLMs and generative AI.
The problem?
Generative AI is built upon patterns of probability rather than facts.
Google is researching the fundamental reasons why news headlines and summaries are generated incorrectly and has developed an evaluation framework called ExHalder. Another example is Bloomberg (subscription required), which has had to issue multiple corrections to summaries generated by AI and LLMs only this past week or so.
Regardless of the weaknesses of using LLMs in search (and they are not without controversy in the world of information retrieval, as Najork alludes to in his 2023 SIGIR presentation) generative AI / generative information retrieval is out of the gate and now represents a fundamental shift in how information is accessed and delivered.
This also has major implications for SEO. Optimizing content to rank in “10 blue links” is different from optimizing for inclusion in an AI-generated summary.
Traffic referral challenges
One big question raised in the presentation is what happens to referral traffic when language models generate answers.
We’ve been seeing this question play out in the form of lawsuits, such Chegg suing Google over AI Overviews. We’ve also heard about many websites of all sizes seeing organic search traffic fall since the launch of AI Overviews, especially for informational queries.
In the “classic” search model, users clicked on links to get information, driving traffic to the websites of brands, creators, and businesses. However, with generative systems, users may get what they need directly from an AI answer without needing to visit a website.
This has been a big source of contention. If AI is trained on “public” content and uses that content to generate responses, how do the original sources get credit or, more importantly, get traffic they can monetize?
This unresolved issue has significant implications for anyone who relies on organic search visibility to drive business results. And as we found out recently, Google seemed to internally view giving traffic to publishers a “necessary evil.”
Najork’s presentation didn’t offer a solution, but this seems to hint at a bleak future for some content creators who can’t adapt to this shift. As Najork put it:
- The pessimistic view: Direct answers reduce referrals to content providers, hurting their ability to monetize.
- The optimistic view: Attribution in direct answers will lead to higher-quality referrals that in aggregate are more valuable.
- The realistic view: Expect diversified business models and revenue streams.
However, we should note that content creation is largely driven by the incentive of search engine-driven traffic, and even a “necessary evil” is “necessary,” so it is more of a challenge to adapt to the new landscape rather than abandon SEO.
Najork also mentioned the important term coined only in 2023 of “delphic costs” by Andre Broder, a distinguished engineer at Google, who also created the well-known A Taxonomy of Web Search. The argument around delphic costs is that the cost to the searcher is greatly reduced by generating answers directly in search results rather than sending the searcher to other resources, and this should be a key objective of search engines.
How will this be achieved and play out? That remains to be seen.
However, we could see as recently as Google’s Search Central event in New York lots of delphic cost savings for searchers in the future-focused presentations.
Expect delphic costs (or similar talk around reducing friction for searchers) and the cost-saving elements of search for users to increasingly influence the communications between Google and SEOs.
SEO vs. GEO
There has been some ongoing and recent debate over semantics among SEO influencers and experts on LinkedIn and elsewhere about whether generative engine optimization (GEO) is simply a new buzzword (and also, how dare we rename SEO!).
I saw a lot of this recently after Christina Adame’s article, How to integrate GEO with SEO, published here on Search Engine Land.
OK. Nobody is renaming SEO.
SEO isn’t GEO.
GEO isn’t SEO. In fact, there is a research paper all about GEO.
Generative (answer) engines aren’t search engines. As Fred Laurent put it to succinctly on LinkedIn:
- “AI Interprets, Search Engines Rank”
This is a key difference to understand. Citations/mentions in AI-generated search are not traditional rankings.
Also, a car isn’t a truck, but both automobiles have engines that can help you get where you want to go.
2023 may be known as the dawn of generative information retrieval, but that doesn’t mean information retrieval is gone. It simply has another facet. This is the way, too, with SEO.
We are in a period of unprecedented change.
Generative information retrieval underlies the new reality of search, but it is still search and information retrieval, but with additional nuance.
In the same way in information retrieval there are those who specialize in recommender systems, indexing, ranking, learning to rank, and natural language processing (NLP) or the front door areas around how search engine users interact with search interfaces, this change in SEO also creates another nuanced area where some will focus and some will generalize.
The core fundamentals of helping users find the right information at the right time remain the same, regardless of the naming convention.
Bottom line: SEO is evolving (again).
If you’re clinging to old SEO playbooks, you could go the way of the dinosaur in the very near future, as Google continues to shift further away from classic search to AI answers.
Note: You can see Najork’s deck on Google Slides. Hat tip to Dawn Anderson for sharing and reviewing this article for accuracy.